The 'Right' Evaluation Tools for Complex Interventions
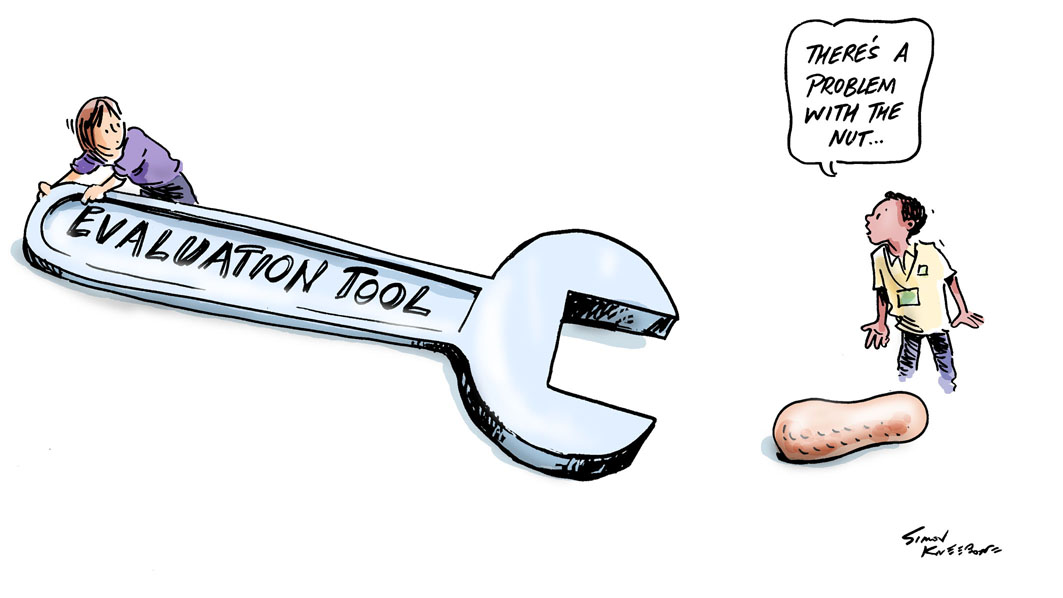
Can you monitor or even evaluate a project for which baseline data was never collected? Or a project for which a proper logical framework was never developed and targets were never set? What about interventions where cause and effect relationships are poorly understood? Or programmes that are implemented in dynamic contexts that make it difficult to predict all the expected outcomes and unintended results seem to emerge on a regular basis?
An increasing number of development projects feature some (or all of the above) characteristics and as such fall into what is called the ‘complex’ category.
Now if you are the programme manager of a ‘complex’ programme you may be scratching your head on how to track implementation or prove impact in the absence of a logical framework, poorly defined indicators, limited baseline data, no set targets and every few months an unintended (and unexpected) thing pops up!
These types of programmes are not readily suited for the conventional evaluation methods. That is, methods that rely on indicators, predetermined objectives and where the change process between cause and effect is known, linear and straightforward. Just as in the cover photo, using traditional monitoring and evaluation methods for complex programmes it is like applying a wrench to peanut. Though the issue to be assessed is a nut, it is not the inappropriate type of nut for a wrench.
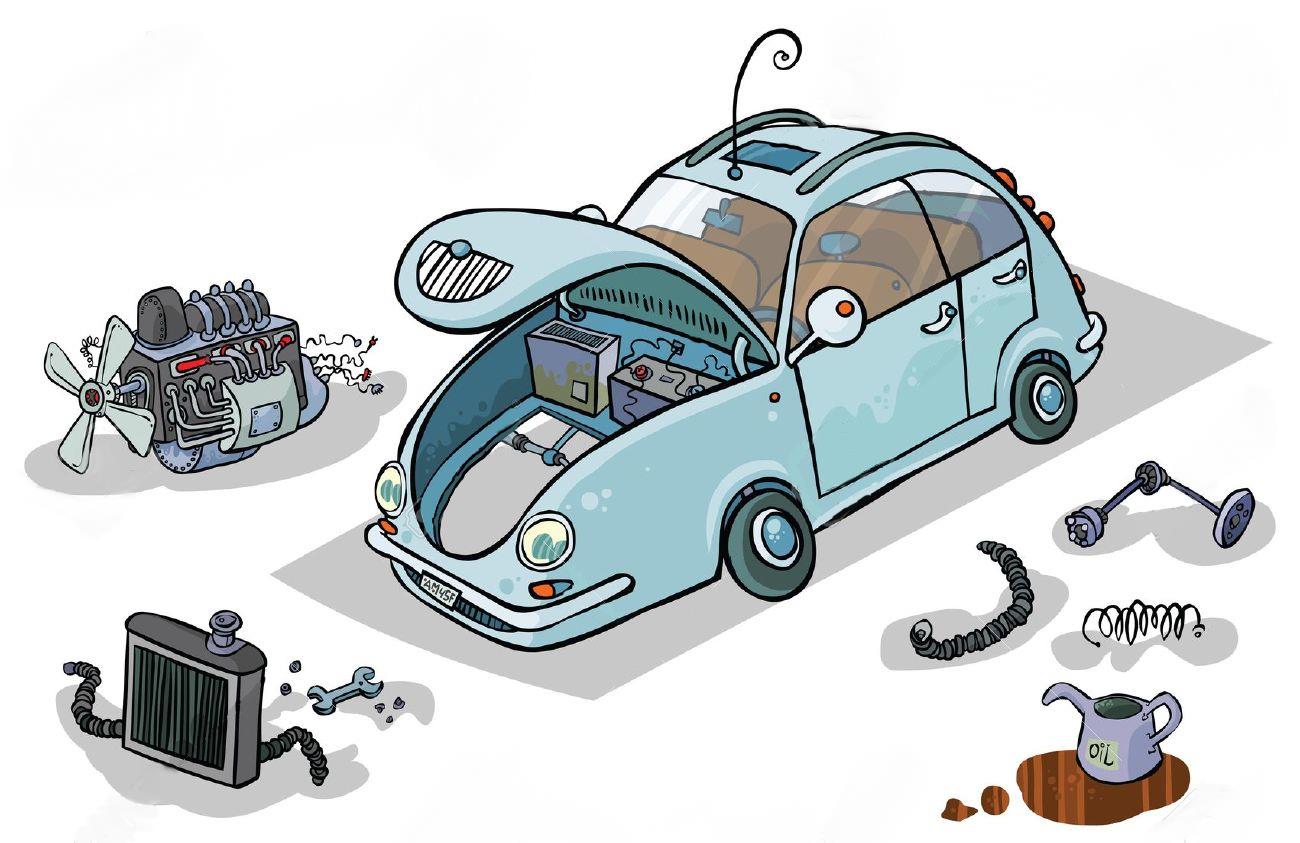
Just like a car without all its parts, it can be hard to monitor and evaluate a programme without 'baseline', 'targets' etc.
Well, luckily there are monitoring and evaluation methods (or ‘nutcracker’ if we are to use the same analogy) that are suited for ‘complex’ (‘peanut’) programmes. These methods don’t rely on predestined indicators, baseline and the like. However, before we delve into a brief description of these methodologies, we should explore in more detail what is meant by the term ‘complex’.
What is a ‘complex’ project of programme ?
To answer this question we refer to the Cynefin Framework. This is an approach to decision-making and knowledge management that was developed in 1999 within IBM. This ‘sense-making’ model has been adopted and applied within the development sector as a part of adaptive management. The Cynefin Framework has five domains:
Obvious: in this domain the relationship between cause and effect is obvious to all. The approach to decision –making under this domain is to Sense- Categorise- Response. Under these conditions we can apply the ‘Best Practise’.
Complicated: in this domain the relationship between cause and effect requires analysis or some other form of investigation and/or the application of expert knowledge. The approach for making decisions is to Sense - Analyze - Respond and we can apply ‘ GoodPractice.
Complex: in this domain, the relationship between cause and effect can only be perceived in retrospect, but not in advance, the approach is to Probe - Sense - Respond and we can sense Emergent Practice.
Chaotic: in this domain there is no relationship between cause and effect at systems level, the approach is to Act - Sense - Respond and we can discover ‘Novel Practice’.
Disorder: in this domain there is the state of not knowing what type of causality exists. In this state of affairs people will revert to their own comfort zone in making a decision. In full use, the Cynefin framework has sub-domains, and the boundary between obvious and chaotic is seen as a catastrophic one: complacency leads to failure.
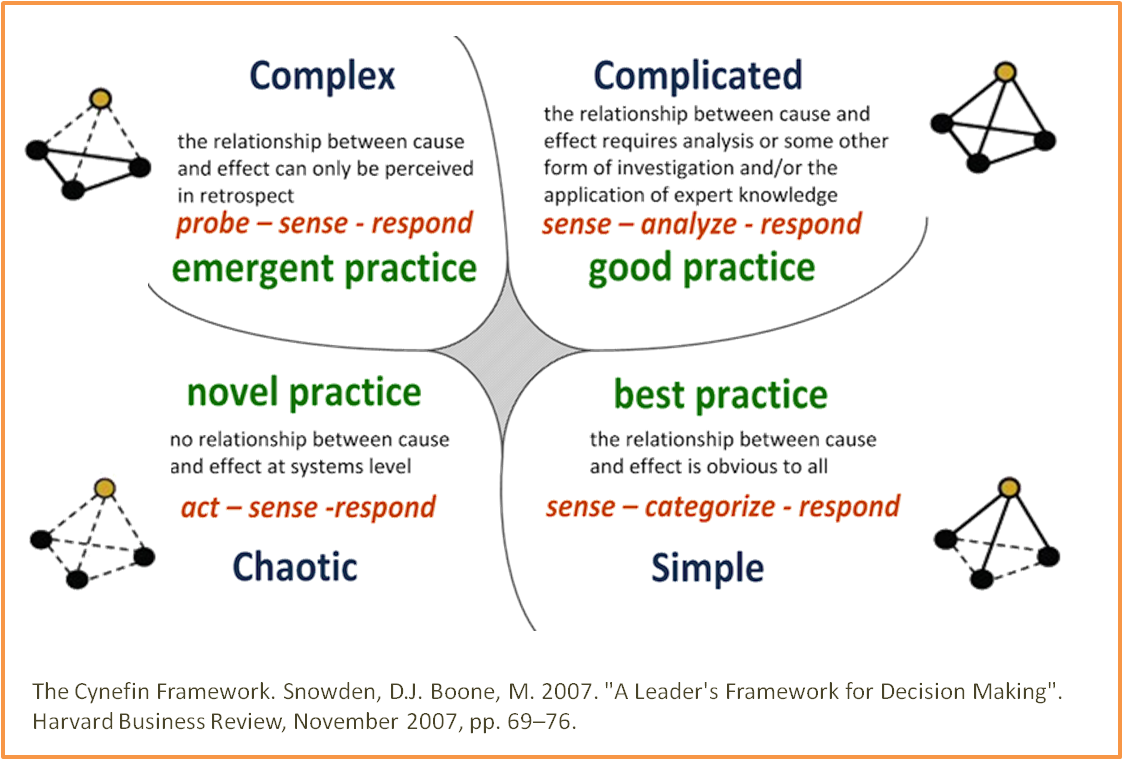
What are ‘complexity-aware’ monitoring and evaluation methods?
As the name suggests, these are methodologies that can be used to gauge the effectiveness of complex interventions. In other words, for projects or programme for which there is not a direct, observable link between the activities of the programme and the observed results. Sometimes it is only at the end of an intervention can one truly see and say what the impact has been.
For example, a lot of interventions that focus on lobby and advocacy fall in this category. At the onset of the programme you hope to influence decision-making. It is only after a period of time spent lobbying can you state in retrospect that it was your actions that led to the enactment of a specific legislation or change in policy.
Sometimes these complex programmes due their nature have indicators that don’t truly measure the success of the programme. After all, how can you conceive of proper indicators or have robust logical frameworks if unintended and unexpected results arise frequently!
Below is a brief overview of three complexity aware monitoring and evaluation methods.
1.Most Significant Change (MSC)
This is a method which seeks to discover results without reference to predetermined objectives, and work backwards to assess the contribution. All results, whether intended or unintended, positive or negative are captured with MSC.
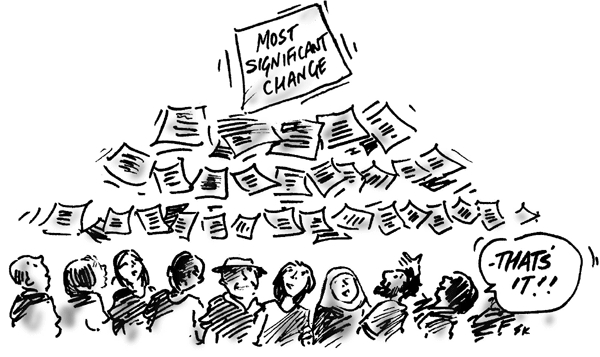
This highly participatory method does not rely predetermined indicators, but rather collects and analyses rich qualitative data in the form of stories.
These stories are collected from all the relevant stakeholders. The question posed to persons are: “During the last period, in your opinion, what was the most significant change that took place for participants in the project?”. Respondents describe both the change and the reasons they consider it significant.
MSC captures differences in development outcomes across sites and time, as well as different perspectives on the same outcomes. MSC is particularly useful when different interpretations of significant change are considered valuable.
If you wish to learn more about MSC, you can join the workshop on 31 October 2023 at 15:00h CEST.
2. Outcomes Harvesting (OH)
Like MSC, OH is a participatory monitoring and evaluation method that enables users to identify, verify, and make sense of outcomes with or without reference to predetermined objectives. OH, however, puts more emphasis than MSC on verification and on identifying and describing contribution ( Discussion Brief, USAID 2016 ).
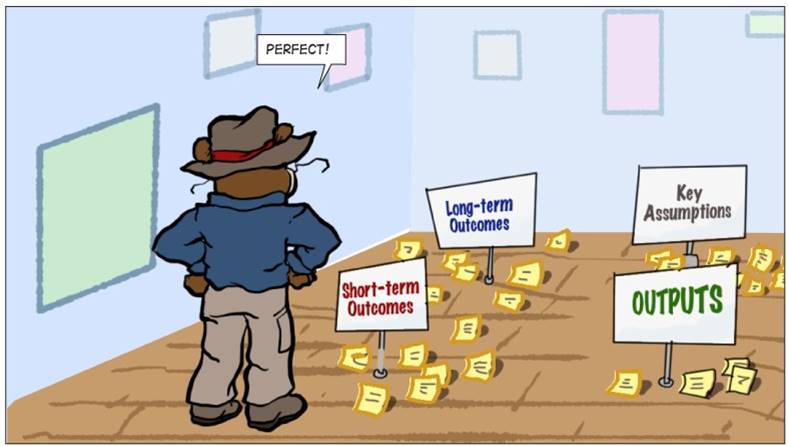
As the name suggests, Outcome Harvesting collects (“harvests”) evidence of what has changed (“outcomes”) and, then, working backwards, determines whether and how an intervention has contributed to these changes.
Data collection is an iterative process that involves reviewing secondary sources, collecting new evidence, drafting outcomes, and engaging with the key informants—the change agents. OH employs systems thinking concepts. The method considers multiple perspectives about who and what has changed, when and where change has occurred, and how the change was influenced.
3. Contribution Tracing (CT)
While both MSC and OH identify, verify and describe the contribution of an intervention to an observed result, CT goes further and allows you to not only test a contribution claim (is it valid or not), but to also determine, quantifiably the level of confidence in contribution claim. The CT developers call it ‘putting a number on it’.
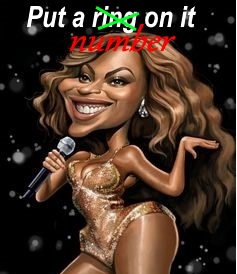
CT is a rigorous quali-quantitative approach that is used in impact evaluations to test the validity of claims. In other words, this approach brings a bit of ‘science’ to determine how confident you can be about a contribution claim.
CT allows you to gather evidence that supports (or is against) your contribution claim. Once all the evidence is gathered it can then be analysed using mathematical formulas (Bayesian updating to be exact). This puts a numerical value on your level of confidence in a particular claim.
For example, after applying the CT approach, an organisation may be able to say with 95% confidence that they are reasonably certain that their lobbying efforts were responsible for the government adopting that specific policy. With CT, there is actually a calculation for how the ‘95%’ was derived.
Conclusion
There are other ‘complexity aware’ monitoring and evaluation methods such as Sentinel Indicators, Stakeholder Feedback and Process Monitoring of Impacts. So don’t panic if your indicators were not formulated in a way to reflect the reality of the programme or if the baseline data is flawed.
The aim of this blog post was to give you, the reader a quick introduction to the 'nutcracker' methods that exist to assess interventions that are less than straightforward (and as such are not suitable to be assessed by the more traditional evaluation methods).
If you are curious to learn more, you can join the workshop on "Integrated Evaluation Methodology: PhotoVoice and Most Significant Change" which takes place on 31 October 2023 at 15:00h CEST.